The Role of Artificial Intelligence to Integrate Robotics in Cost Accounting
Keywords:
Robotic technology in cost accounting, Machine learning ML, Deep learning DL, Internet of things IOT.Abstract
Business operations require robotic implementations to develop enhanced cost accounting systems that handle modern robotic technology cost structures. This paper explores how Artificial Intelligence (AI): Machine Learning (ML), and Deep Learning (DL), together with the Internet of Things (IoT) transform industry robotic sectors through enhanced cost allocation optimization as well as automatic financial reporting and improved operational decision-making mechanisms. A quantitative research design processes data obtained from Turkish industrial and logistics operations which concentrate their examination on IoT-empowered robotic systems. The paper employs ML and DL algorithms for predictive cost modeling and real-time cost optimization. The paper shows that using ML boosts robotic operation forecasts while DL detects maintenance patterns alongside IoT technology that enables quick cost model adjustments leading to more precise financial reporting. Combining these technologies drives extensive cost reduction because ML and DL systems decrease wasteful operations and IoT enables predictive maintenance that reduces equipment shutdowns. Paper findings show that ML, DL alongside IoT technology modifies common cost management systems to deliver improved operational performance, together with better financial precision. The successful implementation of these technologies requires solving three main challenges which include the expense of implementation together with data integration difficulties and transparency-related ethical problems. To achieve maximum cost accounting system benefits from these advanced technologies, businesses should focus on developing flexible solutions alongside resilient governance structures.
References
[1] M. Baker, J. K. Liker, and M. Lee, "Robotics and the cost structure of manufacturing: A framework for cost allocation," J. Manuf. Sci. Eng., vol. 141, no. 2, p. 021015, 2019.
[2] M. Bolliger and A. Ziegler, "Anomaly detection in cost accounting with machine learning algorithms," J. Financial Analytics, vol. 4, no. 1, pp. 15–28, 2020.
[3] J. E. Bessen, "The impact of robotics on labor markets and productivity," Econ. Rev., vol. 103, no. 2, pp. 54–62, 2019.
[4] P. Bryant and H. Thomas, "Future trends in robotic accounting systems: A technology integration perspective," J. Autom. Robot., vol. 34, no. 3, pp. 25–34, 2022.
[5] H. Chen, X. Liu, and Z. Zhang, "Real-time cost optimization in IoT-enabled robotic systems," Int. J. Ind. Eng., vol. 28, no. 5, pp. 1840–1852, 2021.
[6] D. Cowan, M. Goodwin, and S. Hassan, "Assessing financial returns on robotics in manufacturing: A case study using machine learning," J. Bus. Econ., vol. 67, no. 4, pp. 99–110, 2020.
[7] T. H. Davenport and R. Ronanki, "Artificial intelligence for the real world: The promise and peril of automation," Harvard Bus. Rev., vol. 96, no. 3, pp. 108–117, 2018.
[8] X. Feng, Q. Li, and Y. Li, "Optimizing cost allocation with IoT and ML integration: A case study in automated manufacturing," J. Cost Manag., vol. 35, no. 7, pp. 56–68, 2021.
[9] A. Goswami, A. Banerjee, and S. Sengupta, "Using deep learning for predictive maintenance in robotics cost accounting," J. Robot. Autom., vol. 21, no. 3, pp. 110–122, 2022.
[10] S. Karnouskos, "Challenges of integrating IoT in industrial cost accounting systems," J. Ind. Internet, vol. 6, no. 4, pp. 256–268, 2020.
[11] J. Lu, Y. Liu, and M. Zhang, "Leveraging IoT for real-time cost optimization in robotics," Int. J. Robot. Autom., vol. 37, no. 2, pp. 123–135, 2020.
[12] L. Müller, K. Tiede, and S. Krämer, "Integrating IoT with machine learning for cost management in robotics," J. Manuf. Technol. Manag., vol. 33, no. 8, pp. 1080–1095, 2022.
[13] M. Porter, S. Lamb, and P. Bowen, "Machine learning and the automation of cost accounting," J. Account. Technol., vol. 31, no. 1, pp. 24–39, 2020.
[14] F. Sussan, M. Reinders, and A. Koenigsberg, "Ethical implications of AI-driven financial decisions in robotics," J. Ethics Account., vol. 15, no. 2, pp. 78–90, 2022.
[15] X. Zhao, Q. Xu, and Z. Li, "Predicting cost fluctuations in robotic manufacturing with machine learning models," J. Ind. Eng. Manag., vol. 12, no. 2, pp. 100–115, 2019.
[16] X. Zhou, Z. Li, and W. Zhang, "Privacy concerns in IoT-based cost management for robotics: A systematic review," Int. J. Comput. Sci. Eng., vol. 47, no. 6, pp. 221–234, 2021.
[17] E. Brynjolfsson and A. McAfee, The Second Machine Age: Work, Progress, and Prosperity in a Time of Brilliant Technologies, New York, NY, USA: W. W. Norton & Company, 2021.
[18] D. Furió, L. Martínez, and G. Albacete, "A review of accounting issues in robotics integration in manufacturing systems," J. Account. Res., vol. 58, no. 2, pp. 75–92, 2020.
[19] X. He, T. Wang, and Y. Li, "Deep learning for predictive maintenance in robotics: A cost optimization perspective," J. Robot. Autom., vol. 33, no. 4, pp. 25–38, 2021.
[20] J. E. Bessen, Learning by Doing: The Real Connection Between Innovation, Wages, and Wealth, New Haven, CT, USA: Yale Univ. Press, 2019.
[21] [Online]. Available: https://www.postinfographics.com/5-accounting-tech-innovations/
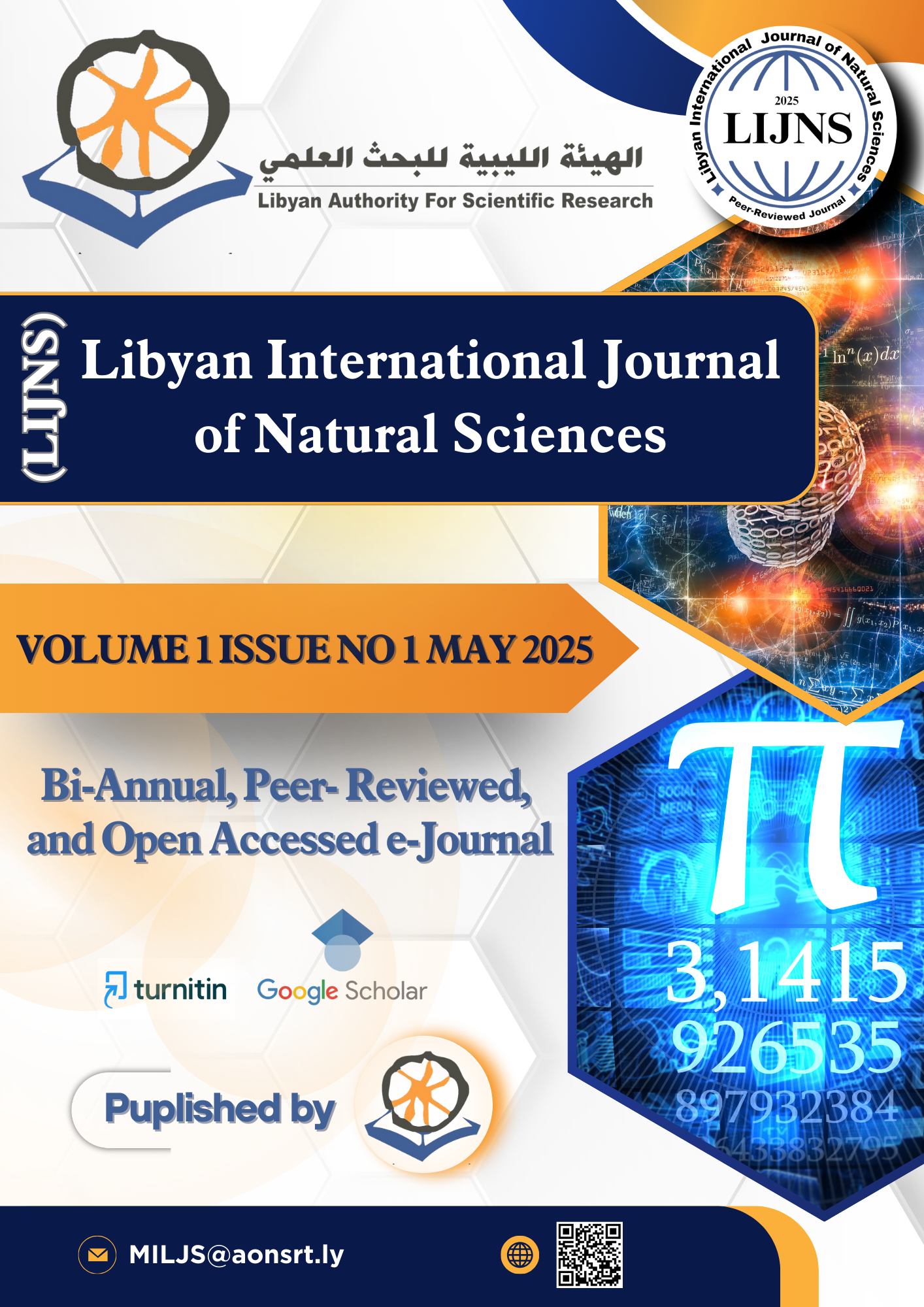
Downloads
Published
How to Cite
Issue
Section
License
Copyright (c) 2025 Libyan Authority for Scientific Research

This work is licensed under a Creative Commons Attribution-NonCommercial-ShareAlike 4.0 International License.